AI-DRIVEN HEALTHCARE DIAGNOSTICS: A REVOLUTIONARY FRONTIER IN MEDICINE
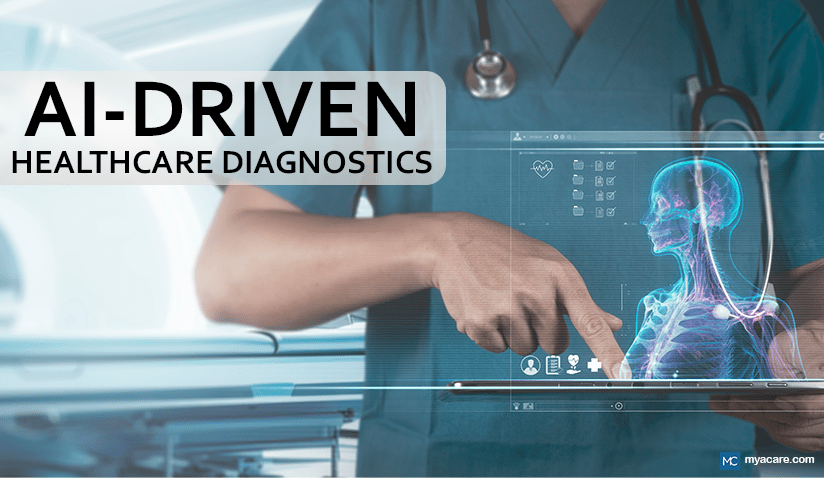
The Evolution of AI in Healthcare
Machine Learning and AI in Health Diagnostics
Applications of AI in Medical Imaging
Healthcare is undergoing an unprecedented transformation thanks to artificial intelligence (AI). One of the most significant areas where AI is making a difference is in medical diagnostics. AI has the potential to improve the accuracy, efficiency, and accessibility of diagnostic processes, as well as to enable early disease detection and enhance personalized medicine.
This blog post covers the evolution, benefits, applications, and challenges of AI in healthcare diagnostics.
The Evolution of AI in Healthcare
AI was originally defined as “the theory and development of computer systems able to perform tasks normally requiring human intelligence, such as visual perception, speech recognition, decision-making, and translation between languages.”
In the 1950s, the first artificial intelligence (AI) systems were developed to carry out simple human jobs that have subsequently been incorporated into computers.[1]
In the field of healthcare, early applications of AI include:
- DENDRAL, which would help chemists quickly identify the chemical formula of an unknown substance from spectrographic data as early as 1965.[2]
- ELIZA, a type of chatbot that mimicked a psychotherapist as a pilot project by Weizenbaum at MIT in 1966.[3]
- Internist-I[4], an experimental diagnostic program that used a knowledge base of over 600 diseases and 4500 symptoms to provide differential diagnosis for internal medicine in the early 70s.
These days, AI can even surpass human intelligence and has managed to outperform students at passing medical exams. Recent advances, such as deep learning, big data, and cloud computing, have enabled AI to achieve remarkable results in healthcare, especially with respect to medical diagnostics.
AI in Medical Diagnostics
AI is now revolutionizing diagnostic tools by analyzing vast amounts of data to provide faster and more accurate integration of patient biometrics, images, medical records, genomic data, and clinical notes. The technology can assist healthcare professionals in making better, swifter decisions that can greatly improve patient outcomes.[5]
AI's ability to manage these voluminous and diverse datasets is transforming medical diagnostics and contributing to more accurate and efficient healthcare outcomes.
Due to these recent developments, some of the world’s most pressing healthcare challenges may well be tended to through AI. With AI diagnostic tools becoming more widely available, diagnosis is made faster, cheaper, and more accurate than what an unskilled or unequipped physician would be able to offer. When combined with telehealth facilities, patients from all over the globe will have access to better healthcare and can receive a diagnosis from anywhere.
Benefits of AI in Healthcare Diagnostics
The advantages of AI in diagnostics are anticipated to significantly raise the standard of healthcare for all. These include:
- Accuracy and Precision: AI algorithms can reduce human errors and biases and provide more consistent and reliable results than human experts. A study by Google showed that an AI system could detect breast cancer from mammograms with higher accuracy and fewer false positives and negatives than radiologists.
- Speed and Efficiency: AI algorithms can process large amounts of data in a fraction of time compared to human experts. IBM proved that an AI system could diagnose acute myeloid leukemia from blood samples in less than four hours compared to the 10 days it took for experts to arrive at the same conclusion.
- Early Detection and Prevention: It is often easy for experts to miss early signs of disease, which can greatly help in disease prevention. AI algorithms can quickly identify the subtle signs or patterns of disease that may be overlooked.
- Personalized Treatment Planning: AI algorithms can provide customized recommendations or suggestions for diagnosis and treatment based on highly personalized patient characteristics, such as genetics, medical history, lifestyle, and preferences. According to study findings, AI could determine from brain scans which therapy option would be most effective for patients with depression.
Machine Learning and AI in Health Diagnostics
All of the above benefits of AI in healthcare are made possible through machine learning.
Artificial intelligence's machine learning field focuses on creating algorithms that can learn from data, spot patterns, make predictions, and improve over time without explicit programming.
When applied to diagnostics, machine learning enables AI to learn from large sets of patient data, identify patterns of disease, and predict outcomes. This specific feature can also be used to create better models of disease used for scientific investigation, allowing for diagnostic guidelines and tools to be developed faster. It can also greatly enhance early disease detection and prevention by quickly identifying the disease path a patient may experience based on their genes and lifestyle factors.
Machine learning is also of great benefit during unknown situations where diagnostics are lacking, such as during a mysterious infectious outbreak or when a new disease is discovered. AI tools can now quickly build a picture of an unknown illness by compounding symptoms alongside differences in genetics, proteins, blood markers, and other parameters to diagnose patients. Similar techniques can be applied to isolating the genetic code of pathogens, which similarly aids in their detection.
Some of the real-world examples of machine learning in medical diagnosis are:
- A mobile app from SkinVision that uses machine learning to analyze skin lesions and quickly assemble a risk assessment for skin cancer.
- GNS Healthcare uses machine learning to discover causal relationships between genes, drugs, and diseases from genomic data.
- The AlphaFold system developed by DeepMind uses machine learning to predict protein structures from amino acid sequences garnered through genomic sequencing.
Applications of AI in Medical Imaging
The greatest advancements of AI in healthcare arguably pertain to medical imaging. Medical imaging is a process that involves capturing internal images of the human body for diagnostic purposes. Imaging can provide vital insights into the structure and function of molecules, cells, tissues, and organs.
Medical imaging devices and techniques improved by AI algorithms include:
- Computed tomography (CT)
- Magnetic resonance imaging (MRI)
- Ultrasound
- X-ray
- Positron emission tomography (PET)
- Optical coherence tomography (OCT)
In the past, these devices were limited in terms of their processing power. While highly accurate, results were still prone to producing poor-quality images that would interfere with diagnosis, especially with respect to moving body parts (e.g., heart or lungs) and deeper internal compartments.
AI has transformed imaging by improving the quality, accuracy, speed, and efficiency of these devices. When applied to these methods, AI can swiftly extract critical information from the results, identify anomalies, measure dimensions, compare data from different modalities or time points, and enhance images, aiding in disease identification.
AI Imaging Techniques and Technologies
The main techniques used by AI technologies to improve imaging are discussed below, alongside examples of these technologies currently being employed in diagnostics.[6]
Image Enhancement and Reconstruction: These days, the best AI-assisted imaging software automatically corrects images, allowing for dramatic improvements in a fraction of the time. Some AI programs are capable of constructing complete images from incomplete data as well, as is commonly seen with basic (fan beam) CT scans that analyze teeth for dental purposes.
Examples of AI-assisted image enhancement technology include:
- DeepMind AI technology can reduce the MRI scanning time by 90%, subsequently enhancing image quality by minimizing the effects of patient movement.
- Subtle Medical made an AI system that can reconstruct PET images from low-dose data and improve image quality and quantification.
Cardiac AI imaging systems are also currently being developed that can take ECG results and use them to improve the quality of real-time imaging. This allows for shots of the heart to be taken in sync with the heartbeat, minimizing distortion.[7]
Segmentation and Detection: Programs now exist that can separate or isolate different regions or objects in images. Anomalies within images can be detected by shape recognition, where AI matches the shape of a growth and highlights them; or more specifically, by assigning labels or masks to pixels or voxels. This helps to improve diagnostic accuracy and allows for the speedy detection of disease entities such as tumors, nodules, or discolorations.
Examples of such features include:
- The Arterys AI system that can segment cardiac and brain MRI images and measure their function.[8]
- Lunit system that detects lung nodules from chest X-rays and CT scans.
Image Classification and Recognition: Following from segmentation and detection of inter-image anomalies, AI can also assign labels, categories, and classifications to images as well as to the objects found within them. This kind of data can be extrapolated from larger databases, allowing for the system to reach a swift diagnosis, as well as to contribute towards databases for future diagnostics.
Examples of classification and recognition technologies include:
- Zebra Medical Vision’s AI system that can classify chest X-rays into 40 common findings.
- The IDx-DR system that can recognize diabetic retinopathy from fundus images (pictures of the retina) and provide a diagnosis.
AI in Diagnostics and the Patient-Doctor Relationship
Short consultations, budget constraints, highly detailed patient information, and differences between the doctor and patient can all pose challenges to reaching an accurate and swift diagnosis. These are especially problematic when seeing a specialist or new GP for the first time. AI can greatly enhance these key considerations by saving time and consolidating patient record information.
Patient records are documents that contain information about a patient’s medical history, symptoms, diagnosis, treatment, medications, allergies, test results, vital signs, and other relevant data. They are often unstructured and uploaded as doctor notes that contain lots of information. This helps physicians to keep track of the patient’s case history and can provide insights into a patient’s health status and risk factors for diseases or conditions.
When dealing with patient records, AI utilizes natural language processing (NLP) techniques to structure patient records as well as analyze them for patient’s emotions and opinions, disease entities and terminology, useful patterns or patient’s habits, medical questions, and physician’s answers. Doctors often do not have the time to go through their notes in as much detail and tend to fall short of patients’ needs and expectations as a result.[9]
AI can enhance the patient-doctor relationship by offering practical suggestions to both the patient and doctor, as well as pinpoint problem areas based on this information. For example, AI may answer a patient’s questions better and give advice phrased in a way more sensitive to the patient’s emotions or opinions.
It may also help to identify long-term patterns of disease or patient behaviors that may contribute towards a current diagnosis and help to improve a personalized treatment plan.
Some natural language processing AI systems already in use include:
- DeepTag- This scans patient photographs and assimilates patient data instantly from facial features. It can also be used to assign medical codes to patient records by analyzing the data in them, which aids both diagnosis and billing.[10]
- cTAKES- This structures doctor notes and provides valuable insights and suggestions to help streamline consultation and general practice.
AI in Disease Diagnosis
As highlighted throughout this article, AI has moved diagnostics into an era of personalized medicine. It helps not only to diagnose specific diseases but also to diagnose inter-patient differences, profiles, and disease subtypes as well.
AI can help integrate and analyze multiple sources of data, such as clinical notes, laboratory tests, imaging studies, genomic data, proteomic data, metabolomic data, and microbiome data, to provide a holistic view of a patient’s health and disease status. AI can also help discover new biomarkers, pathways, targets, or drugs for diagnosis and treatment.
Specific diseases and medical conditions are starting to be diagnosed at astounding speeds with much greater accuracy. This is particularly pertinent in the fields of cancer, cardiovascular conditions, diabetes, neurological disorders, infectious diseases, and rare diseases. AI technologies have also improved diagnostic research and guidelines, screening, consultations, patient-to-doctor feedback, and awareness of critical events, such as strokes or heart attacks, through real-time alert systems.[11]
Advantages and Disadvantages of AI in Healthcare Diagnostics
The process of weighing the benefits and drawbacks of AI in medical diagnostics is difficult and complex, and necessitates careful consideration.
Advantages
AI has many advantages for healthcare diagnostics, such as:
- Improving Outcomes: The quality of healthcare services benefits from AI by enabling more precise diagnoses, faster and more efficient diagnostic processes, enhanced early disease detection and prevention, and personalized treatment planning.
- Cutting Costs: AI can help reduce the costs and resources of healthcare services by optimizing equipment and facilities, reducing the need for unnecessary or redundant tests or procedures, and minimizing the risks of errors, complications, or adverse events.
- Increasing Access: Access and availability of healthcare services are improved by AI due to enhanced remote diagnostics. This allows providers to reach populations without adequate access to healthcare and minimizes the problems faced in areas lacking skilled practitioners.
Disadvantages
In comparison to the advantages, the disadvantages of AI are relatively few. These include:
- Technical Difficulties: AI systems may be susceptible to hardware failures, software bugs, or network disruptions, which may affect their functionality or performance from time to time. They may also require very specialized equipment to run, depending on the complexity of the tasks they perform.
- Ethical Concerns: Making use of AI systems raises many ethical concerns. AI is prone to making use of inaccurate or biased data, which may be susceptible to misuse or security breaches that can jeopardize patient privacy. It is also not fully transparent about how it reaches its conclusions yet, although better software is likely to overcome this problem.
- Legal Issues: Since AI is not a true entity, it may be difficult to hold AI liable or accountable for failures or problems that emerge from its use. This may require new laws, policies, or standards to solve.
Future Trends and Innovations
AI is constantly evolving and innovating in healthcare diagnostics. Some of the future trends and innovations that may shape the field are:
- Telemedicine and AI-powered remote diagnostics that can provide quality care for patients in rural and remote areas or in situations where physical contact is not possible or desirable.
- AI Integration in Wearable Devices and Sensors that can monitor and diagnose various health conditions in real-time. These devices can send updates to healthcare providers, contribute to global databases, and offer useful alerts or tailored recommendations to users.
- Precision Medicine Technologies are being developed that can provide highly specific diagnostic information based on a patient’s genetic and molecular profiles. This can help to tailor treatments and therapies as well.
- Electronic Health Records that can store and analyze large amounts of patient data with AI assistance, quickly providing greater insights for diagnosis and treatment.
Conclusion
AI is transforming the field of healthcare diagnostics in unprecedented ways. It has the potential to improve the accuracy, efficiency, and accessibility of diagnostic processes, as well as to enable early disease detection and enhance personalized medicine. However, AI also poses some challenges and limitations that require careful consideration. The future of AI in healthcare diagnostics is promising and exciting, as it will bring new opportunities and innovations that can benefit patients, healthcare professionals, and society at large.
To search for the best doctors and healthcare providers worldwide, please use the Mya Care search engine.
Sources:
- [1] https://sitn.hms.harvard.edu/flash/2017/history-artificial-intelligence/
- [2] https://www.britannica.com/technology/DENDRAL
- [3] https://dl.acm.org/doi/10.1145/365153.365168
- [4] https://www.nejm.org/doi/full/10.1056/NEJM198208193070803
- [5] https://www.ncbi.nlm.nih.gov/pmc/articles/PMC7325854/
- [6] https://www.ncbi.nlm.nih.gov/pmc/articles/PMC7055429/
- [7] https://physicsworld.com/a/acrobeat-mitigates-cardiac-motion-during-imaging/
- [8] https://www.fiercebiotech.com/medtech/arterys-lands-fda-go-ahead-add-new-modules-cardiac-mri-reading-ai
- [9] https://www.foreseemed.com/natural-language-processing-in-healthcare
- [10] https://www.avma.org/javma-news/2019-06-15/predict-my-codes